Modeling to Define Policy
by C. Nataraj, PhD, professor, Mechanical Engineering, and director, Villanova Center for the Analytics of Dynamic Systems
This essay was written in March 2020 at the start of the pandemic:
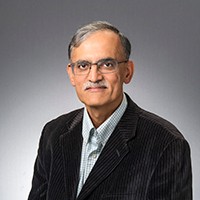
Dr. C. Nataraj
Natural catastrophes are a lot scarier than the ones we engender (for example, a car accident) because of the lack of precise understanding we have about them and the cloud of uncertainty that seems to surround them. Their magnitude, impact and the predictability of future evolution all seem uncertain, which further leads to a sense of helplessness as these events seem to be out of our control while our engineered lives seem to be mostly controllable.
Mankind has always tried to deal with a natural disaster by attempting to develop some insight so that we can predict how bad it is, how much bigger it will get, when it may happen again, and how many people—and who—are going to be affected by it. Even the motion of the planets and the eclipses were frightening to our ancestors, so they dealt with it by developing very approximate models, which were nevertheless astonishingly accurate and led to future developments in mathematics and physics that we now take for granted. In the 21st century, we routinely use modeling as the basis of predicting most natural phenomena including weather-related events such as storms and floods.
The Coronavirus epidemic is horrific in its possible implications, and the news media is overrun with talk about “models”—some trust the models that are predicting millions of deaths, and others believe it is all a “hoax” perpetrated by the liberals and alarmists, scientists among them. So, are the models right or wrong, and can we trust them? The answer, of course, is—it depends.
First, let us note that a so-called computer model is largely a mathematical model that is implemented on a computer and is an approximate (and abstract) representation of reality. Reality is indeed very complex, and so we must make approximations and assumptions to derive a model. This model still has unknown quantities that we call parameters, which are unknown. We use real measured data to get best estimates of the parameters in order to improve predictions. Also, since they have stochasticity embedded in them, there will always be a band of uncertainty so that the best they can do is predict probable scenarios. Finally, the computational tools integrate measured data and employ a variety of tools (including AI) to make the predictions.
Let us understand this in the context of the epidemiological models of the virus. These models are not new; in fact, they have been around for about a hundred years, and they have been honed by back-testing against past epidemics. The one making the news (in March 2020) is the one developed at Imperial College that apparently was scary enough to change the approach taken by our federal government. There are some key parameters in this model that were estimated using high quality data and robust quantitative analysis. These include the current reproduction number, incubation period, the number of asymptomatic cases, fatality ratios, etc. Note that the accuracy of prediction is highly dependent on the choice of these numerical values. Hence, the real value of the model is not for exact predictions, but to do a ‘what-if’ kind of analysis. For example, what if we did not do anything? We would have over 2 million deaths in the US. Suppose the model is off in its predictions, and “only” 500,000 die; is that even remotely acceptable to us? But note that the model also says that, with proper measures such as social distancing and quarantining infected cases, we can bring it down by orders of magnitude. This then is the real value of the model which guides us on what we can do and suggests how our actions can dramatically alter the future course of the disease. Hence it is important for us to not panic over such predictions but to use them as sobering numbers that should convince us of the potential seriousness of the situation, and the need for all of us to do our bit to reduce the transmission.
Models such as this are never perfect and can fall short in many ways. They can fail to include some important aspect of the problem that we don’t know about; they may have limited range of applicability (size of population, time range, etc.); or, we may be off in the values of the parameters. In fact, such has been the case for almost all scientific models we have developed over the past two millennia. Scientists don’t give up; we simply develop better models and improve our predictions. Short of relying on the farmer’s almanac or horoscopic charts, the epidemiological models we have today are the best tools with which to predict the course of this dreadful virus and to guide policy actions. Let us trust the scientists!
To learn more about modelling policy mathematically, refer to this publication for which Dr. Nataraj was the chief author:
Kwuimy, C. A. K.; Nazari, F.; Jiao, X.; Rohani, P. & Nataraj, C. (2020), 'Nonlinear dynamic analysis of an epidemiological model for COVID-19 including public behavior and government action', Nonlinear Dynamics. https://rdcu.be/b6dX2